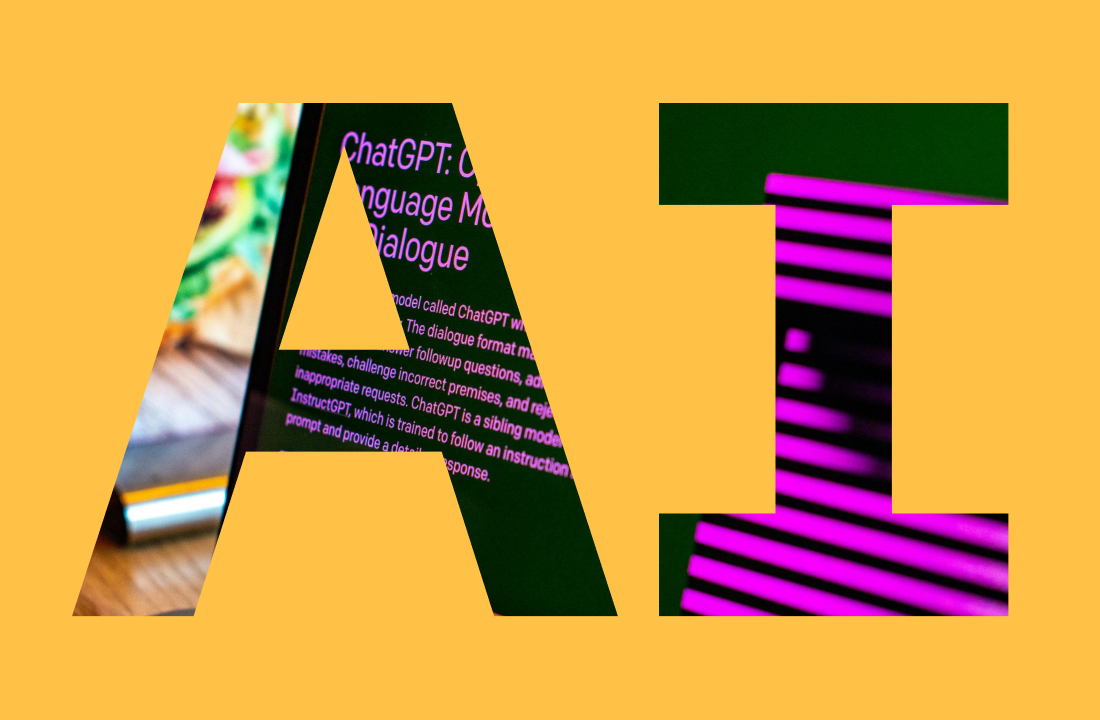
AI (artificial intelligence) by ui42 Recommendation tool based on artificial intelligence for eshops
Recommendation tool based on artificial intelligence for online shops
Recommendation tool is a proven way for online shops to up-sell and cross-sell products or services. However, when online shops want to expand their portfolio, manual clicking or random generated recommended products stop being sufficient. Solution might lie in artificial intelligence. Automation (system itself can substitute its administrators) but mainly higher numbers of important metrics would make any client happy. And that is exactly what we at ui42 have been very good at - thanks to our recommendation tool.
Particularities of Slovak e-commerce market
Artificial intelligence in e-commerce environments has been successfully used by big world players such as Youtube, Alibaba or Netflix. It enables them to display personalised content to visitors without them having to share any data. These projects, however, use big amounts of data to create datasets and train models in order to reach sought-after results. Our initial hypothesis then was:
Problems many online shops need to tackle
- a need to find the suitable tool for additional sales for an online shop with a big assortment
- offer of relevant products/services that potential clients might want to purchase
- automation of up-sell/cross-sell activities
- conversions improvement - click-through rate/sale
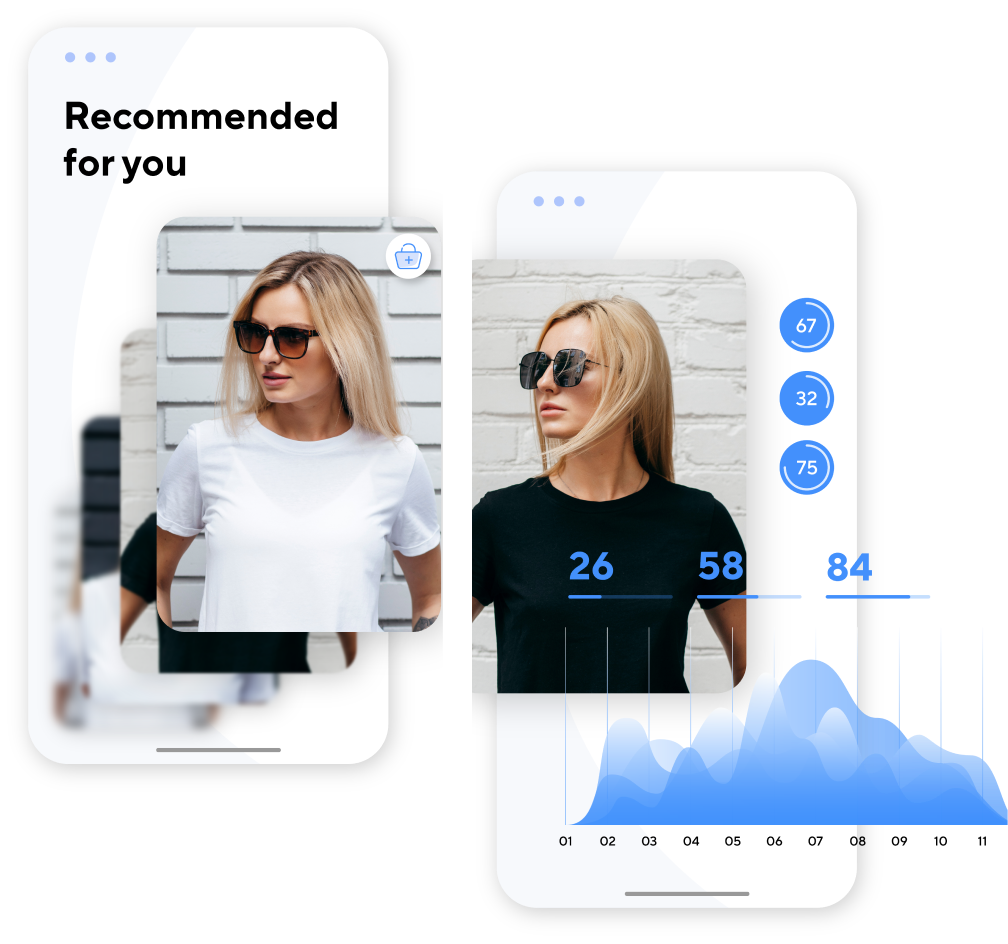
Is artificial intelligence suitable for online shops which process considerably smaller amounts of data?
Solution: Recommendation tool by ui42
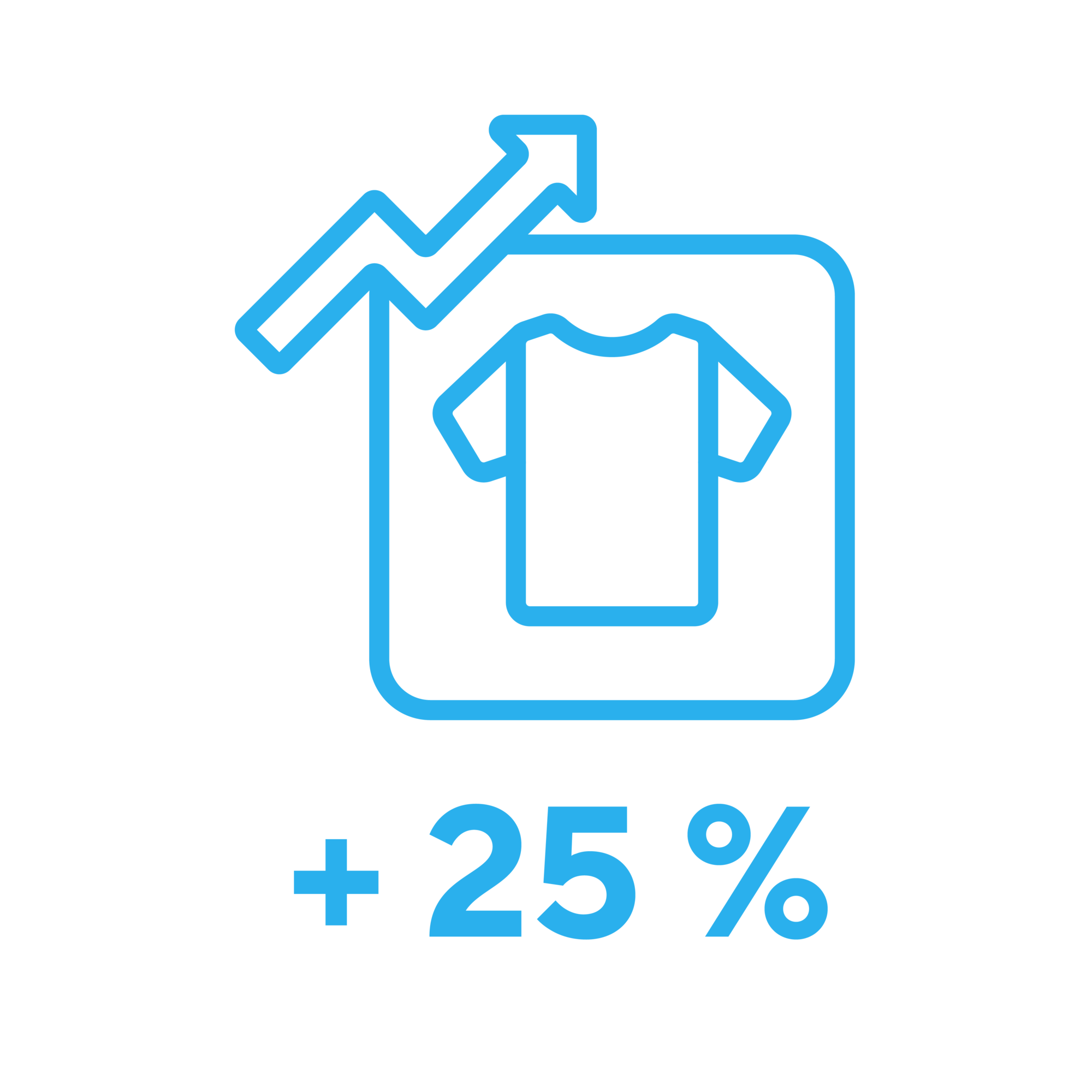
Increases revenue in product details by 25% per visit
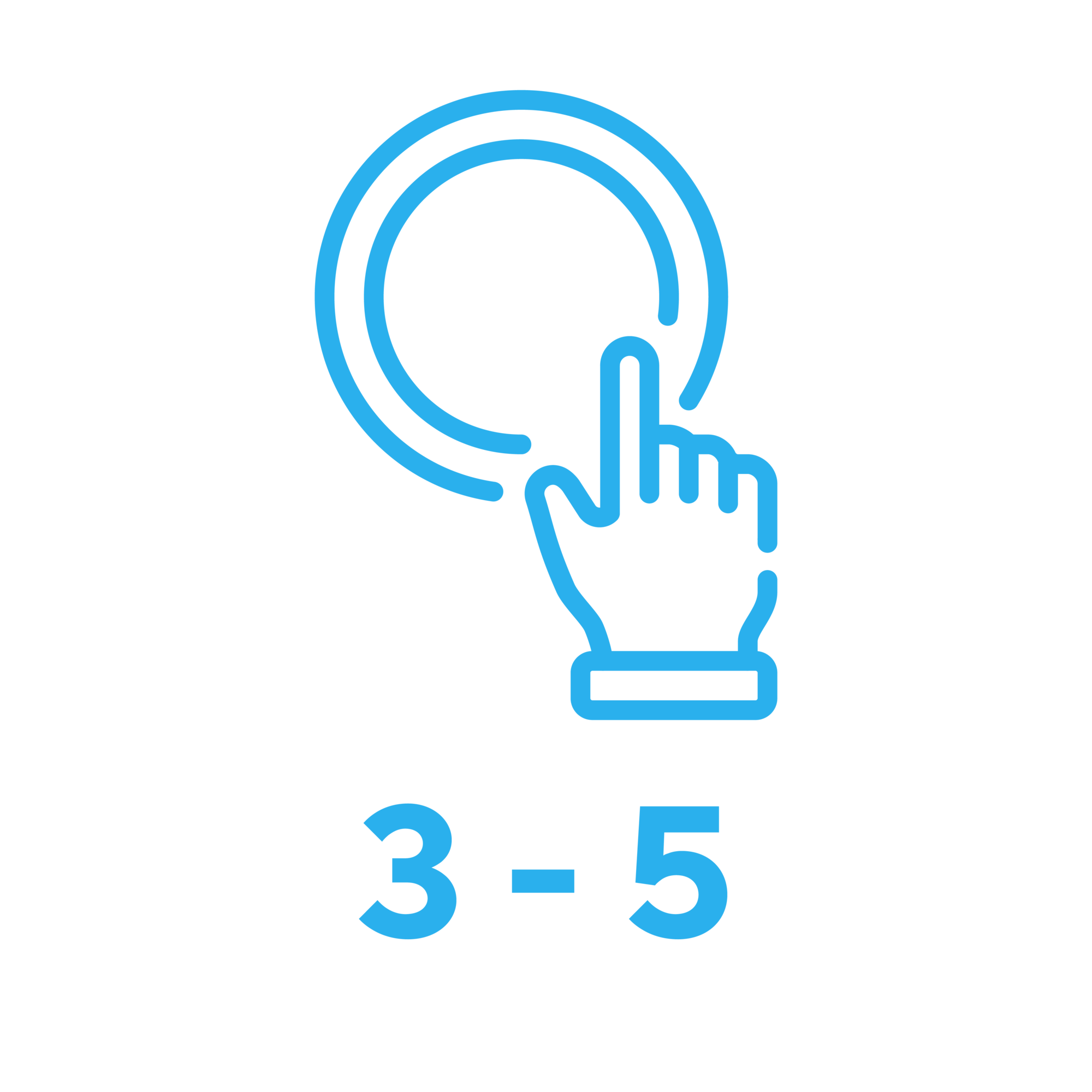
Increases CTR – Click Through Rate 3-5 times compared to the original solution
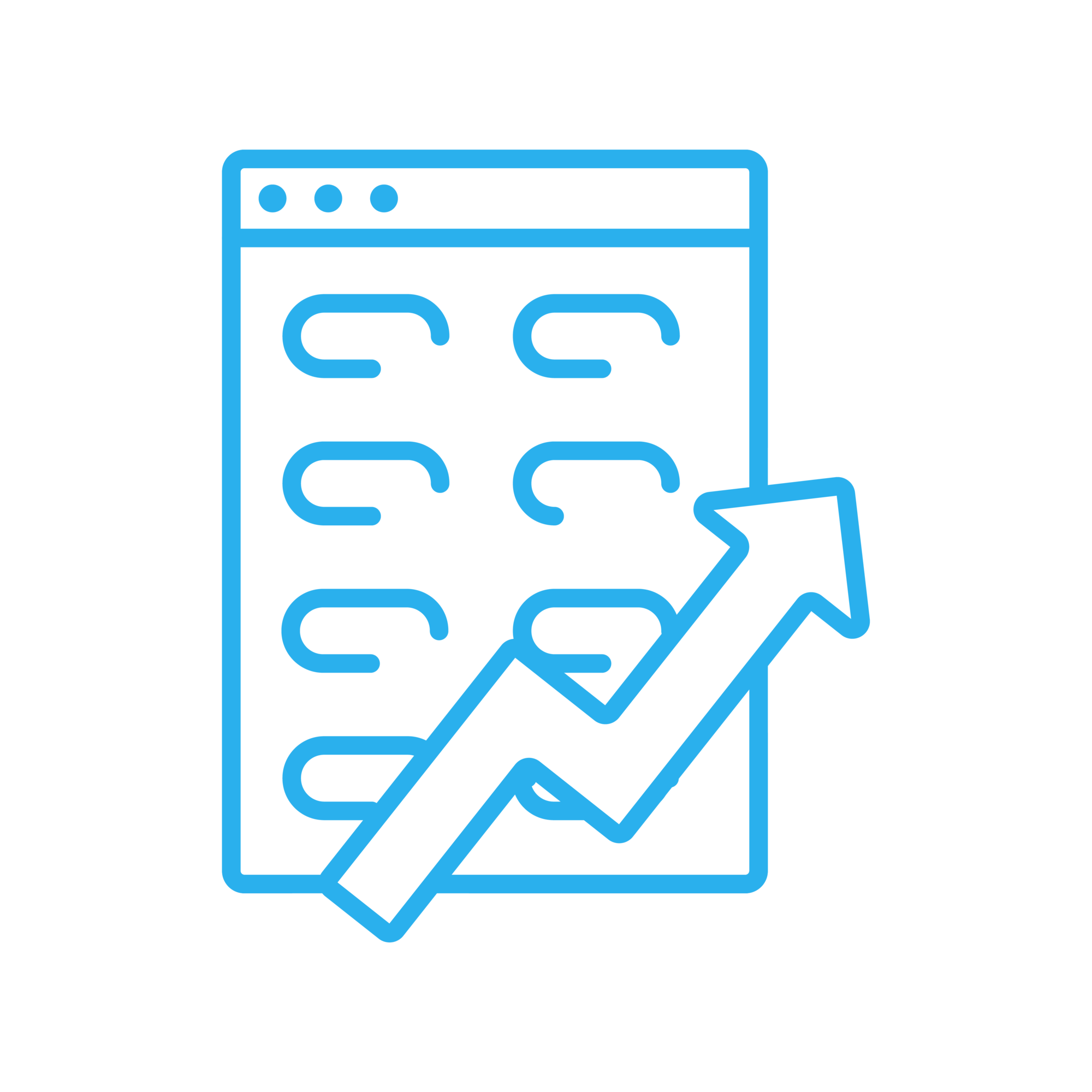
Increases number of activities within single visit
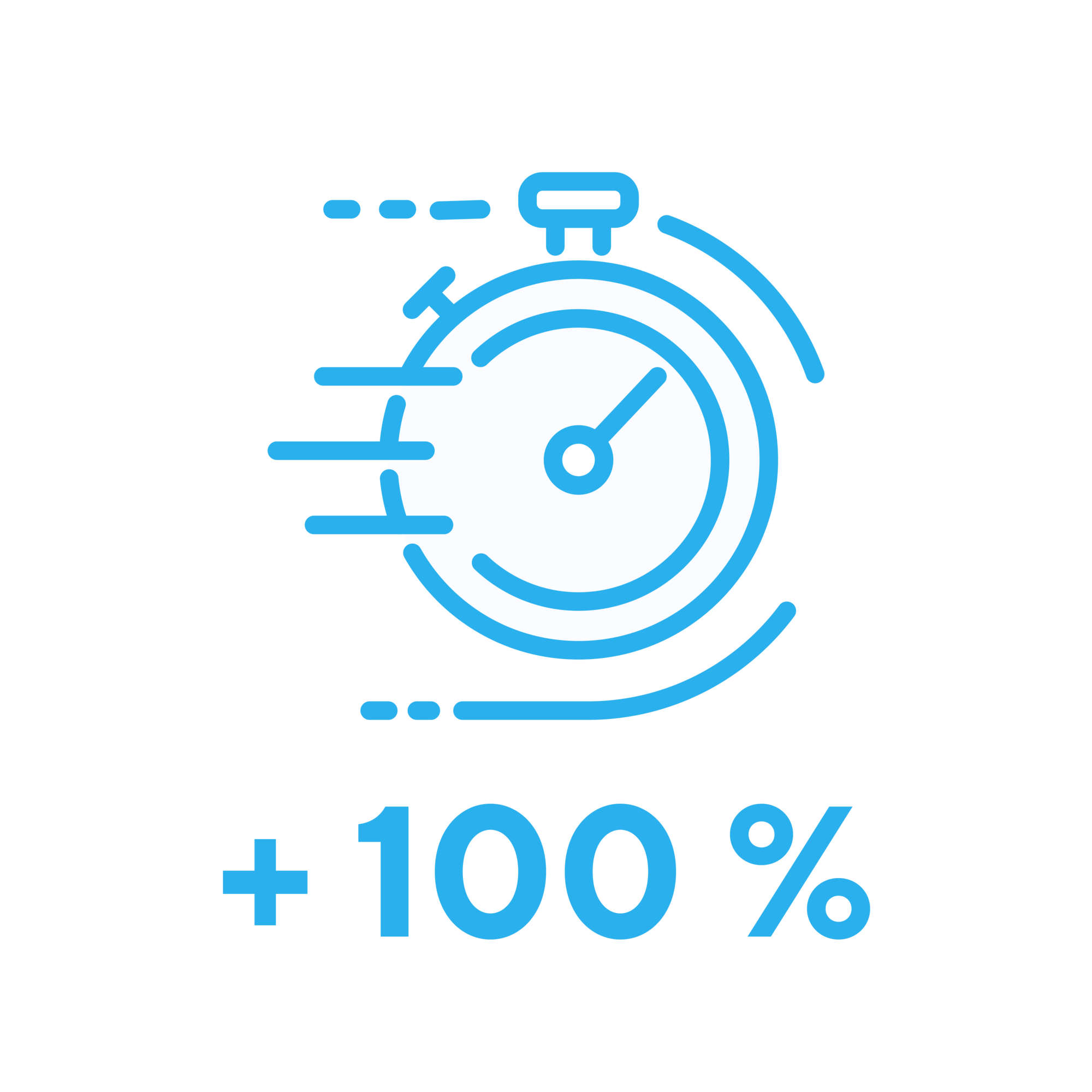
Increases time spent on the website by 100%
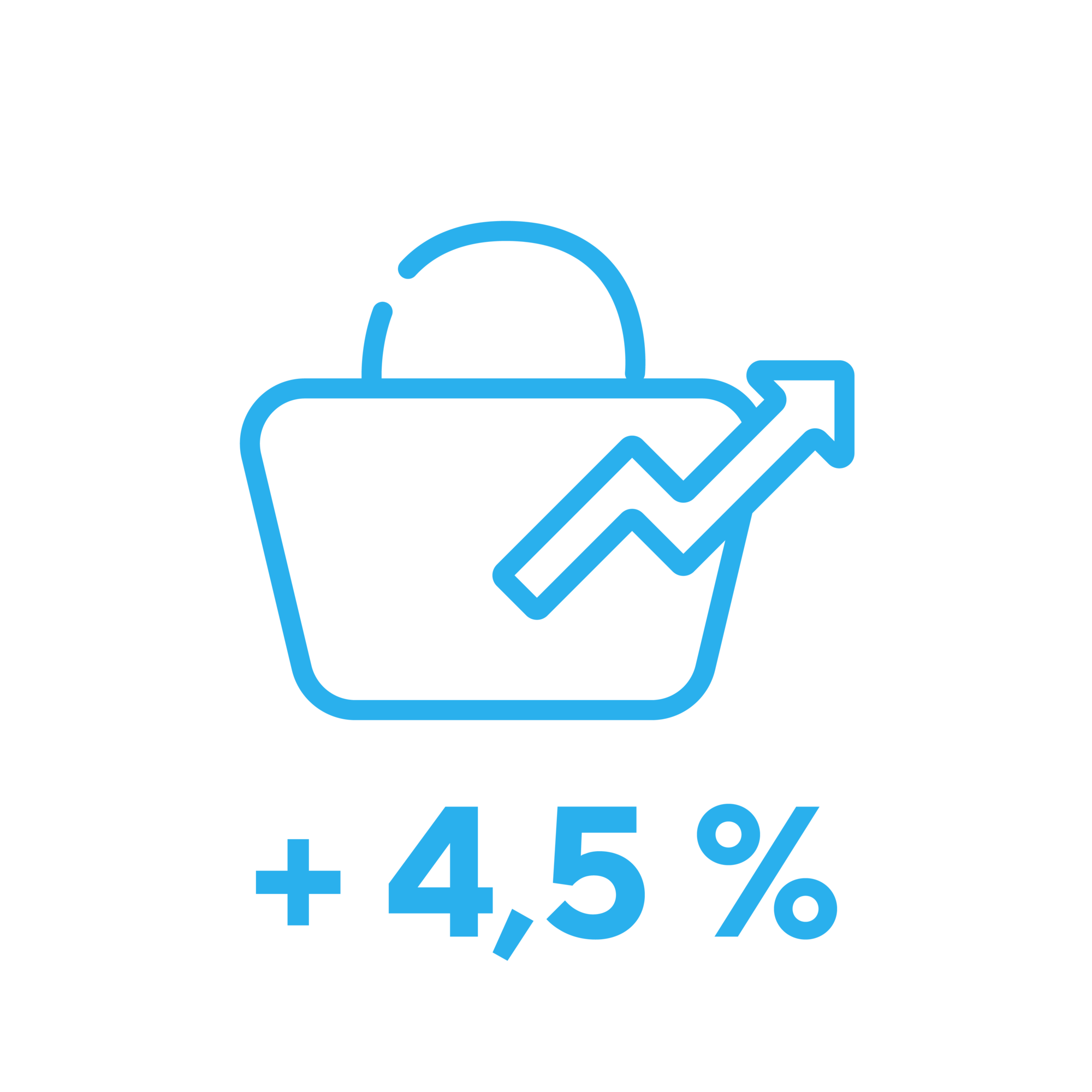
Increases revenue in the basket by 4,5 % per visit
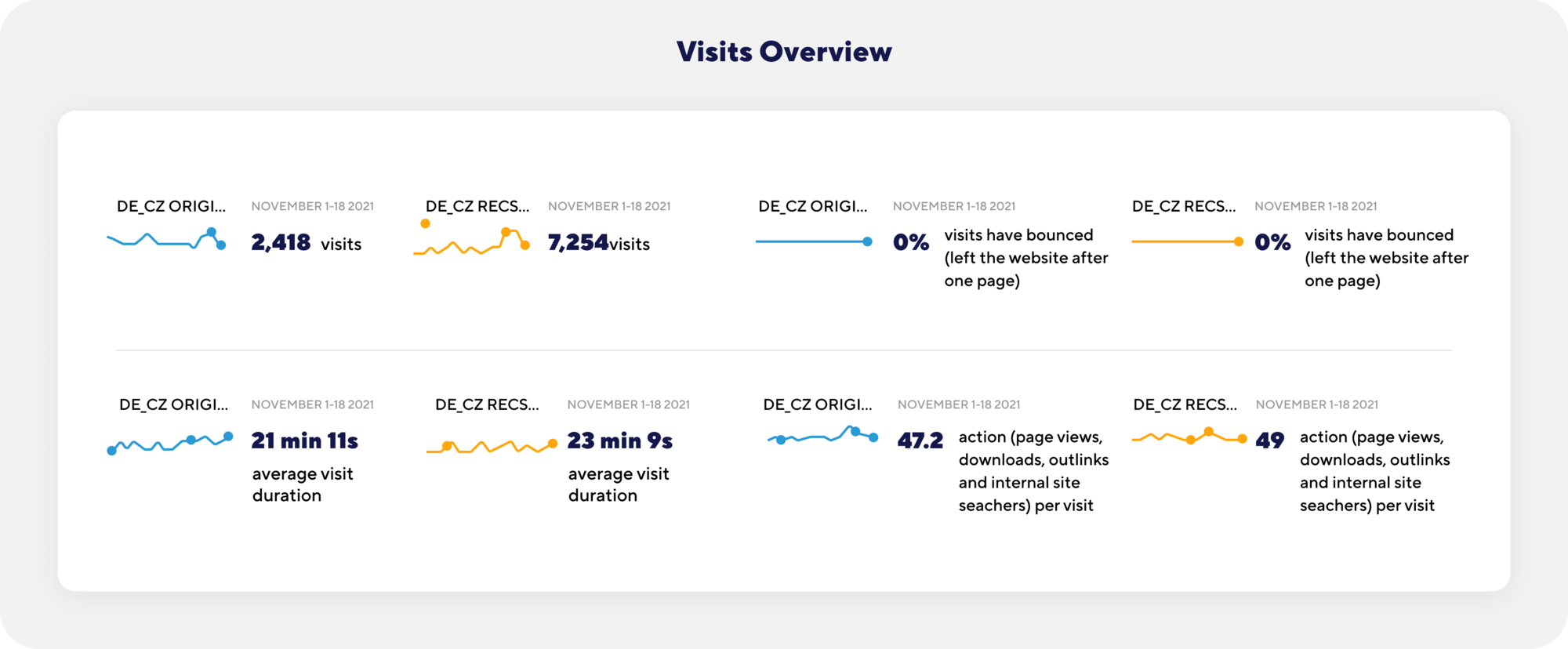
How does it all work?
We have already mentioned our ui42 experiments with artificial intelligence on our blog. ML – machine learning as an artificial intelligence field can use collected data and use a programmed algorithm to create a statistical model. This model can then be implemented in website applications (recommendations, virtual assistant, spam, etc). Thanks to accessibility of faster graphics processing units – GPU and our own server which we called a Centrální mozek lidstva – CML (Central Brain of Humankind), we can train the collected data on our own models and consequently apply them in the outside world. As a bonus, you also get A/B testing that we are so well-known for. It is this testing that enables us to relevantly evaluate the merits of our pilot project focused on Artificial Intelligence - recommending tool.
Benefits of Chatbot
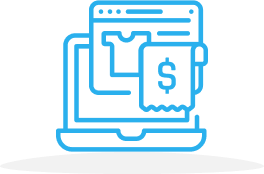
Small and medium-sized online shops
with a potential for performance growth
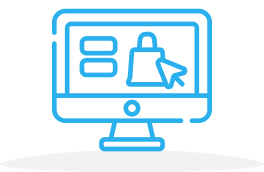
Online shops with a large amount of products
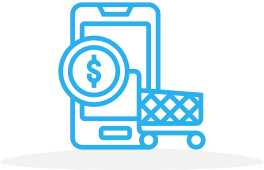
Online shops with a large amount of orders (data)
which need a functional cross-sell
or up-sell tool
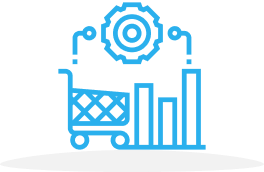
Websites with content that makes product pairing using attributes difficult
Requirements from an online shop
The basic requirement for using artificial intelligence on the website’s background is quality data. Most importantly it is the amount of data our clients can collect and we help to collect them in such a way that our clients can also access them. Many products that are difficult to pair up due to unclear attributes (photograph, colour, use, etc) can become obstacles to a usual way of creating a list of recommended products. For an online shop with a broad portfolio, it is practically impossible to create a cross-selling tool using manual clicking. Recommendation tools however can fully automatically, without any other inputs whatsoever, display an offer which catches the online shop’s visitor’s eye because it is tailor-made to his needs.
Solution by ui42 versus usual recommendation tools
When the time comes for automation, the client has multiple options and we are proud to say that the Recommendation System made by ui42 has achieved better results for “usual solutions” than other algorithm based recommendation tools. The tests predict conversions increasing by 80% when compared to the results achieved with a recommendation tool that has become favourite of many Slovak online shops with large assortment.
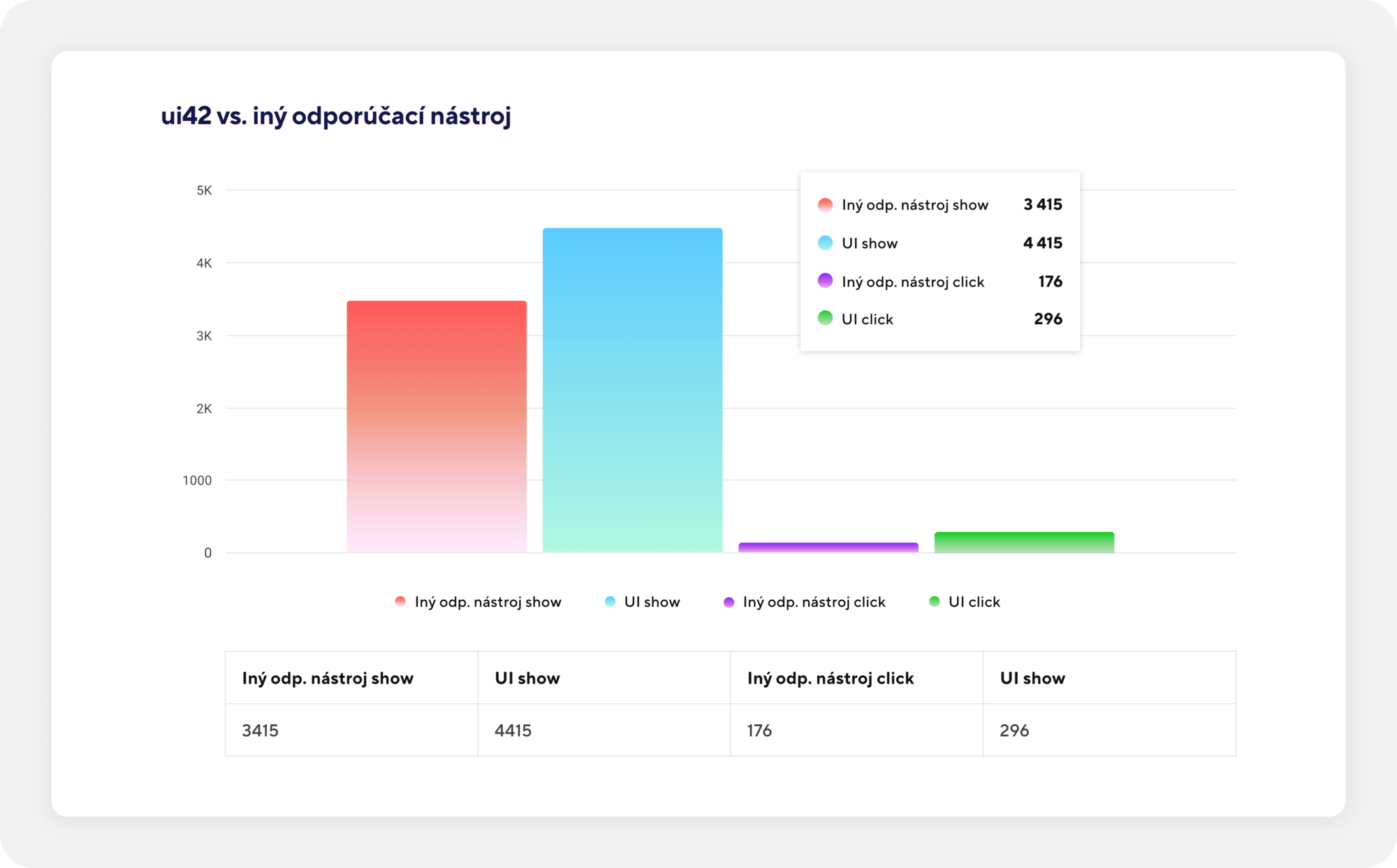
Implementation steps
Data Collection
For machine learning, data collection is key. ui42 run their own analytics tool on their own server. It is an alternative to Google Analytics which was no longer sufficient for the needs of recommendation tool by ui42. The main reason is that the recommending system can also access transactional data (not only aggregated) and moreover, all data is fully under our control.
Monitoring, measuring and evaluating of success
It is important to constantly monitor and check the data, as any error in setting up the recommendation tool would make it necessary to start the data collection from the very beginning. We use A/B Testing - in some cases also A/B/C Testing - to verify the results and to be able to compare our solution to the original one but also its future possible adjustments.
Preparing datasets and training models
Transactional non-aggregated data need to be sorted into datasets where they fall into categories by sessions, which consequently enter neural network.
Example:
For our client Dedoles, we worked with the following data:
- Product views
- Ordered products
- Products put into cart
Results for a recommendation tool on the product website
Recommendation tool by ui42 has been successfully implemented in Dedoles’ online shop hidden behind “You might also like” in the product’s detail.
Input details: Product views > 80 000 000 records
Goal: To recommend related products according to customers’ preferences
Observed metrics: CTR (Click Through Rate) oproti pôvodnému odporúčaniu
Result: In its three markets (SK, CZ and DE) our client Dedoles reached 3-5x higher click through rate compared to the previous recommendation
Verification: A-B Testing
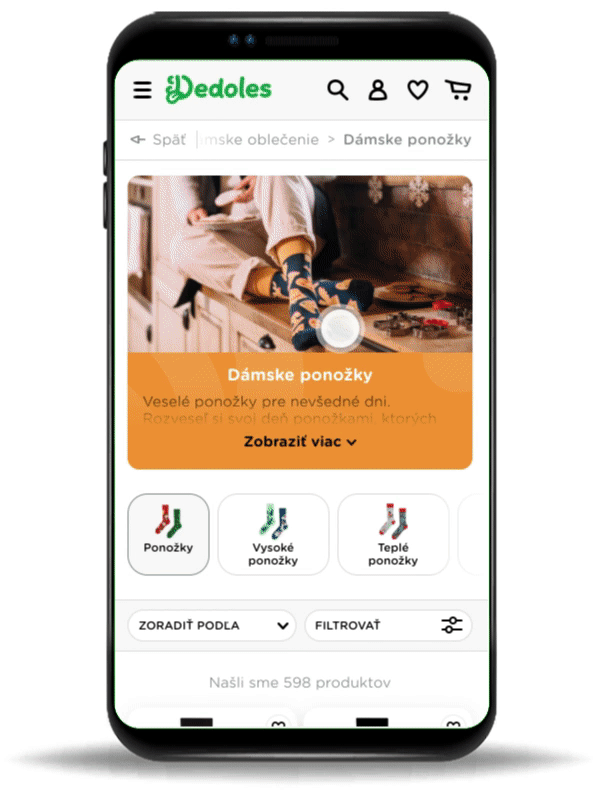
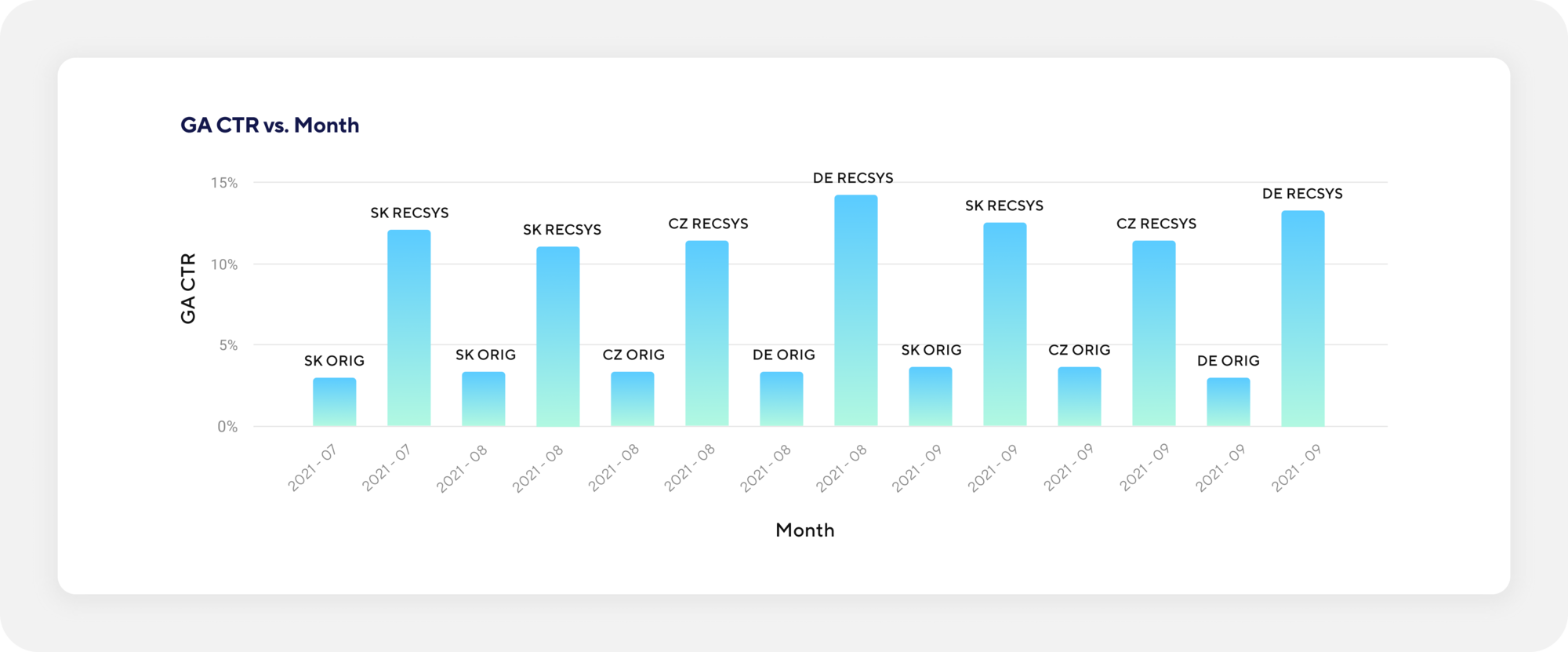
Results for the recommendation tool in shopping cart
Another possible location for the recommendation tool by ui42 is recommendations in the basket, which is based on a different algorithm than the product detail.
Input details: Sales from the basket (orders dataset) > 4 000 000 records
Goal: To recommend products the customer might want to be interested in buying based on the basket’s content
Observed metrics: Order value
Result: Increase in average order value
Verification: A-B-C Testing
Where can you use Recommendation by ui42?
- Category page and product placement optimisation (sub-categories) within it
- Home page
- And others

And we are only warming up…
After our first successful steps, we are going to continue experimenting with artificial intelligence. New recommendation tool has helped grow many of our clients and at the same time can automate processes in their e-commerce projects with amazing results. If you’d like to see whether your online shop can grow at this rate, do not hesitate to contact us and we will be more than glad to help.
Contact us
Are you interested in a recommendation tool that will level-up your online business?
-
Boris Henezi
Sales Executive
-
Samuel Štassel
Sales Executive