How recommendation systems transform information intake: Personalization, FOMO, and information bubbles
The evolution of news consumption has truly been revolutionary and swift. The days when newspapers and evening news broadcasts were our main sources of information are long gone. Nowadays, the power belongs to us, the readers and consumers of news, which allows us to tailor our own news channels through social media, apps, and increasingly sophisticated recommendation systems. These advancements and innovations have not only changed how we access news but also how we interact with it.
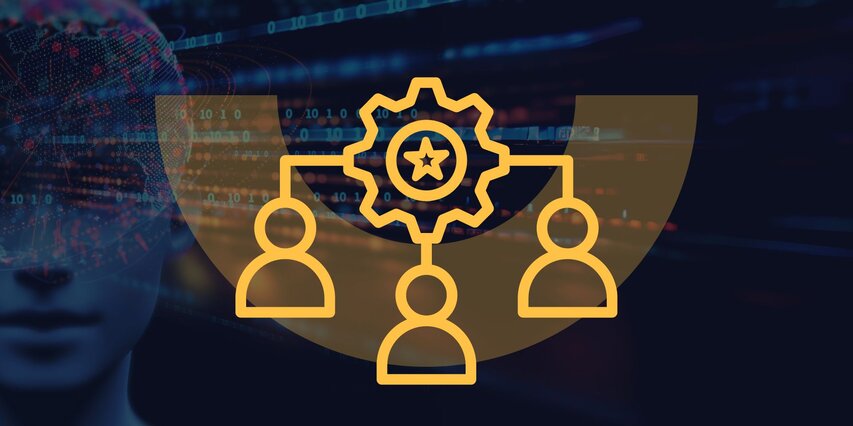
```html
Personalization, the Battle for Attention, and the Quantity of Content
The digital era has brought an abundance of information. Every day, an enormous amount of articles, editorials, videos, and multimedia content is generated and created. According to the latest research, the amount of information we receive daily far exceeds what we can process. This information overload requires a system that can sift through communication noise and effectively deliver relevant content. In the past, only a few key sources of information dominated the media environment. Today, countless communication channels, profiles, news outlets compete for our attention, each presenting its seemingly "unique approach" to current events. A well-designed recommendation system acts as a guide in the crowd of information, helping us navigate the chaos of information overload and find content that truly interests us. Without such systems, we may feel overwhelmed and consequently stop following and seeking (even) relevant information.
Crème de la crème of personalization - for you page #fyp
A significant element of modern recommendation systems is the personalized "For You" pages. Imagine arriving at your preferred news website and immediately encountering a selection of articles tailored to your interests. This feature eliminates the need for lengthy searches and browsing content that may not be relevant to you. Whether you're interested in technology, global politics, sports, or lifestyle trends, the "For You Page" ensures that you spend less time searching for content and directly receive content that genuinely interests you.
Furthermore, personalized "For You" pages use advanced algorithms to continuously improve their recommendations. These algorithms analyze your previous behavior, including articles read, time spent on the site, and interactions with social media, to provide you with even more accurate and relevant recommendations. The result is a dynamic and seamless user experience that adapts to your changing interests and preferences.
For you page sites also have the ability to include content that you might not have searched for yourself but could interest you. This broadens your horizons and allows you to discover new topics and perspectives, thereby enhancing your overall experience and knowledge. In this way, personalized recommendation systems not only increase your satisfaction but also promote a broader and deeper approach to information.
FOMO - Fear of Missing Out
The vast amount of available information can cause readers to experience FOMO (Fear of Missing Out) - the fear of missing out on important updates or interesting events. This phenomenon is particularly pronounced in the digital era, as the constant influx of news and updates can be overwhelming and lead to a feeling that there is always something we are missing at any given moment.
Recommendation systems alleviate this modern anxiety or fear by presenting us with the most relevant content tailored specifically for each individual. They use advanced algorithms to analyze our interests and preferences, allowing them to filter through the vast amount of available news and tailor the selection of the most important and interesting articles precisely for us. Recommendation systems are capable of continuously learning and adapting to our changing preferences. As our interests evolve over time, the systems update their recommendations, ensuring we always have access to the most current and relevant information. In this way, they help not only to reduce FOMO but also to maintain an overview of the topics that are most important to us.
Moreover, recommendation systems can be designed to introduce us to various perspectives and expanding information that we might otherwise overlook. In this way, they not only mitigate FOMO but also support our overall awareness and enable us to better understand complex topics from multiple angles. Ultimately, these systems help us feel more informed and less overwhelmed by the vast amount of available information.
Discovering New Content
Recommendation systems improve the discovery of new content by offering us information and content that we would not actively seek out, but has the potential to interest us. In this way, recommendation systems extend our time spent consuming content. This extended engagement time is beneficial not only for the user, who gains a richer and more diverse experience, but also for platforms that profit from a higher retention rate.
Recommendation systems also expose us to new topics, opinions, and perspectives, thereby deepening and enriching our overall experience and knowledge. For example, if the system exposes us to articles about cultural events, scientific discoveries, or social issues that we might otherwise have overlooked, it broadens our horizons and supports our overall awareness. Ultimately, recommendation systems play a key role in shaping our information "diet" and experiences from content consumption. Their ability to continuously offer new and interesting content ensures that we never feel stagnant or bored, quite the opposite. In this way, they contribute to enriching our daily lives and the development of our knowledge and interests.
Reader Experience
A seamless, personalized experience often leads to higher reader satisfaction. When readers feel that a news website or profile understands their preferences, it is more likely that they will become its loyal visitors. This sense of understanding and personalization creates a strong bond between the user and the platform, increasing the chances of long-term loyalty. This engagement is reflected in longer time spent on the site, a higher number of shared articles, and an overall increase in visibility and credibility of the website or profile. When readers feel satisfied and have positive experiences, they are more willing to share content on their social networks, thereby extending the reach of the website. Loyal readers often contribute valuable feedback and interactions, which can help the platform continuously improve its services and content. Their positive reviews and recommendations can increase the credibility and authority of the site in the eyes of the wider public. Ultimately, satisfied and engaged readers contribute to the lasting success and growth of news websites and profiles.
What's behind it?
1. Named-entity recognition (NER)
A key component of recommendation systems is named-entity recognition (NER), which falls under NLP - Natural Language Processing. NLP is a field of artificial intelligence that enables computers to understand, create, and manipulate human language. Natural language processing has the ability to query data using text or voice in natural language, opening the door to many advanced features and applications.
NER is a technique that identifies and categorizes key entities in text. These entities include people, organizations, places, dates, and other categories that are relevant to understanding the context and meaning of the text. For example, if an article mentions "Elon Musk" and "SpaceX," the system recognizes these entities and may suggest other articles related to space exploration or technological innovations, matching the reader's interests. NER works based on machine learning algorithms that learn to identify and categorize entities from large data sets. These algorithms use statistical and linguistic models to recognize patterns in text. This process involves extracting keywords and phrases, analyzing them, and then assigning the correct category. As a result, the system can not only recognize specific names and places but also understand their relationships and context.
Implementing NER in recommendation systems has several advantages.
- First, it allows for the personalization of content based on the specific interests of the reader. If the system knows that a reader is interested in a particular person or topic, it can offer relevant articles and information.
- Second, NER improves the system's ability to recognize and respond to current events and trends. If a new significant event suddenly appears in the media, the system can quickly identify it and adjust recommendations accordingly.
An important part of utilizing NER is also its ability to analyze large amounts of text in a short time, which is essential for news portals and other information platforms that process huge volumes of data daily. In this way, these systems can provide readers with current and relevant information in real-time.
In addition, NER can be combined with other NLP technologies, such as sentiment analysis, which evaluates the emotional tone of the text. This allows for even more precise customization of content according to the reader's preferences and moods, thereby increasing their engagement and satisfaction.
Ultimately, named-entity recognition represents a cornerstone for modern recommendation systems that strive to provide personalized and relevant experiences for every reader. Integrating NER into these systems ensures that readers receive content that not only informs but also interests and inspires.
Properly implemented NER technology is therefore essential for the successful provision of personalized recommendations and maintaining a high level of reader satisfaction.
2. Machine learning algorithms
Recommendation systems use complex algorithms that include various techniques, such as collaborative filtering and content-based filtering, to analyze past behavior and preferences of readers.
- Collaborative filtering is based on analyzing the preferences of multiple users with similar interests. This algorithm examines the behavior patterns of several readers and makes recommendations for individuals based on that. For example, if two readers regularly read similar articles, the system may assume that articles that interested one of them will also be relevant to the other.
- Content-based filtering, on the other hand, focuses on the characteristics of the articles themselves. This approach analyzes texts, themes, and keywords in articles that the reader prefers, and then recommends other articles with similar characteristics. By combining both approaches, systems can provide very accurate and relevant recommendations.
These algorithms also take into account various engagement metrics, such as the time spent reading an article, the frequency of interactions with certain types of content, and activity on social media, such as shares or comments. In this way, machine learning algorithms can identify patterns and predict what content will likely interest the reader in the future. This allows for more personalized and targeted recommendations, which increase user satisfaction and engagement.
3. Real-time adaptation
One of the outstanding features of modern recommendation systems is their ability to adapt in real-time. This ability allows systems to instantly respond to reader interactions with content, thereby fine-tuning suggestions and ensuring they remain relevant. As readers read, comment, or share articles, the system continuously analyzes these activities and adjusts recommendations according to current preferences.
This dynamic adaptability is possible thanks to advanced machine learning technologies and the continuous analysis of large volumes of data. For example, if a reader shifts their focus from technology news to sports news, the system quickly records this change in behavior and adjusts its recommendations to better suit the reader's new interests. In addition, real-time adaptation allows systems to respond to sudden changes in the broader context, such as current events or trends. If, for example, a significant news story emerges, the system can immediately increase the visibility of related articles, ensuring that readers are informed about the latest and most important events.
This ability to constantly adapt ensures that recommendations are always up-to-date and relevant, leading to a higher level of satisfaction and engagement among readers. Real-time adaptation thus plays a key role in providing a smooth and personalized user experience, tailored to the ever-changing needs and interests of users.
What are the challenges in the collaboration of artificial intelligence and journalism?
Filter bubbles
Filter bubbles pose a risk to awareness, as readers are exposed only to information that supports their existing views and biases. This effect can reduce the ability of individuals to think critically and be open to new and different opinions.
Privacy concerns
Collecting data to provide personalized recommendations raises many questions regarding the privacy of readers and content consumers. Profiles, websites, and especially news portals must be transparent about data collection, what they collect, and how they use it. They must ensure compliance with ethical principles, rules, and regulations. This includes adhering to regulations such as the General Data Protection Regulation (GDPR) in the European Union, which sets strict rules for the collection, processing, and storage of personal data. Compliance with such regulations is not only a legal obligation but also a moral imperative to protect user rights.
Algorithmic bias
Algorithms, that power these recommendation systems, are not infallible. They can sometimes incorrectly reinforce biases present in the data on which they were trained. Continuous evaluation and refinement are key to minimizing biases and ensuring fair and accurate recommendations.
Conclusion
Perfect personalization is the music of the future
Recommendation systems are not just a trendy accessory for news sites – they are an essential tool in today's era of information saturation. By creating personalized "fyp" pages and using sophisticated technologies such as named-entity recognition and machine learning algorithms, they improve the reading experience and ensure that the audience always finds content that resonates. With great power comes great responsibility. When news organizations implement these systems, we must consider filter bubbles, privacy concerns, and potential algorithmic biases.
For us as readers, the era of personalized news promises a more interesting, relevant, and enjoyable way to stay informed in an increasingly complex world. So, the next time you find an article on your "For you page" that seems tailor-made for you, know that it's the result of a combination of cutting-edge technology and careful content selection.
If you've read this far, you've surely caught many reasons why to apply recommendation systems to your website. Are you constantly wondering how to increase the efficiency and performance of your e-shop, as well as customer loyalty? You don't have to be an information media or news outlet, recommendation systems are used in every industry. Personalize recommended products to each visitor according to their preferences and shopping behavior with artificial intelligence and turn them into a satisfied, loyal customer. Do not hesitate to contact us, we will be happy to advise you on implementing AI and recommendation systems. Read about how you can implement AI in customer reviews.
```